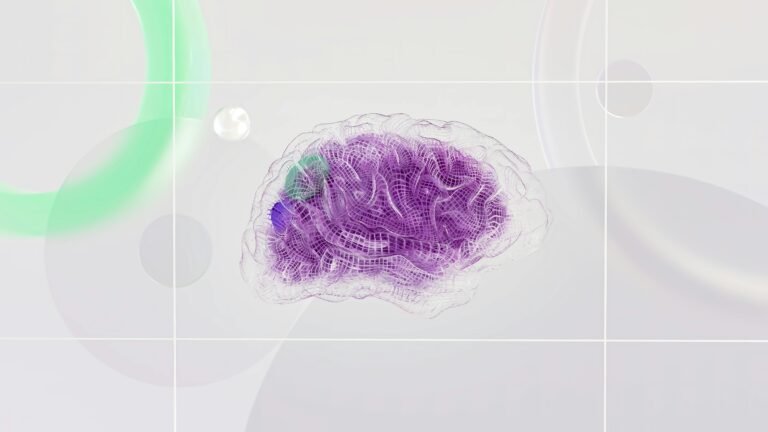
Neuro-symbolic AI stands out as a unique form of artificial intelligence, harnessing the strengths of both symbolic and neural AI architectures.
This AI model can model cognition, learning, and reason, effectively overcoming each of its limitations.
Neuro-symbolic AI: The optimal combination of symbolic AI and neural networks
Neural networks’ competencies in processing large-scale unstructured data are combined with symbolic AI’s efficacy in managing structured knowledge to create neuro-symbolic AI.
This combination enhances the model’s overall efficacy and proficiency in a variety of tasks, thereby fostering confidence in its capabilities.
It is fundamentally a fusion of reasoning in intelligence and neural networks.
Symbolic AI, which has existed since the 1950s, is the most suitable option for tasks requiring comprehensible reasoning, as it processes information using rules and logic.
In contrast, neural networks, a subset of machine learning inspired by the human brain, are particularly adept at identifying patterns and generating predictions when presented with significant data.
Neuro-symbolic AI combines the interpretability and rule-based reasoning of AI with networks’ adaptability and learning capabilities.
Neuro-symbolic AI is a truly interdisciplinary field, merging the critical component of deep learning, neural networks, with the techniques of symbolic reasoning. This broad and deep integration underscores the far-reaching impact of neuro-symbolic AI, making the audience feel the breadth and depth of its influence.
This hybrid approach is designed to bridge the divide between symbolic reasoning and statistical learning, allowing machines to reason symbolically and leverage the robust pattern recognition capabilities of neural networks.
Researchers attempted to integrate symbols into robotics to replicate human behavior. This rule-based symbolic Artificial General Intelligence (AI) necessitated explicitly integrating human knowledge and behavioral guidelines into computer programs.
Distinction between Symbolic AI and Neuro AI
Symbols are essential for communication, influencing our thought processes and reasoning, and improving human intelligence. To comprehend the world, humans establish internal symbolic representations and norms for interacting with it based on logic.
Rule-based AI significantly influences symbolic AI. Symbolic AI, also known as rule-based AI or classical AI, utilizes a symbolic representation of knowledge, such as ontologies or logic, to perform reasoning tasks.
A human can easily understand and articulate the reasoning of symbolic AI, which employs explicit rules and algorithms to solve problems and make decisions.
Symbolic AI is predicated on the human capacity to comprehend the world by establishing symbolic connections and representations.
These symbolic representations establish the standards for designating concepts and capturing everyday knowledge. These systems employ symbols and principles to represent knowledge and execute reasoning.
This implies that to clarify a concept to a symbolic AI system, a Symbolic AI Engineer and Researcher must explicitly provide all pertinent information and principles the AI can use to make a precise identification.
Data is a critical component of neural networks.
The purpose of neural network models is to identify patterns, learn from data, and produce predictions.
Neural networks are composed of interconnected nodes or neurons arranged in layers. These nodes modify their connections according to the computed data. Neural networks are particularly proficient in managing data, which encompasses natural language, audio files, and images.
The “neuro” component pertains to deep learning neural networks driven by the human brain’s ability to compete.
Neural Networks are a type of machine learning inspired by the structure and functionality of the human brain. They utilize artificial neurons, a vast network of interconnected structures, to identify patterns in data and make predictions.
Neural networks are proficient in managing complex and unstructured data, such as speech and images. They can also develop the capacity to perform tasks with a high degree of precision, such as image recognition and natural language processing.
Data is the driving force behind neural networks, as they learn from examples to recognize patterns in language or imagery.
This is why neural networks are so effective at recognizing patterns in language or imagery. Nevertheless, a neural network requires hundreds of examples to identify an object or understand a sentence that contains an unfamiliar word, whereas we only require one or two.
The neural network’s algorithm is initially trained on many images over time instead of focusing on specific pixel patterns, such as edges, as symbolic AI would.
Upon encountering a new image, deep neural networks subsequently construct a model that generates a probability among all potential predictions, thereby accomplishing precise image recognition. Deep neural networks have substantially improved machines’ capacity to perform complex translations into multiple languages and natural language processing.
The purpose of neural network models is to identify patterns, learn from data, and produce predictions.
Neural networks are composed of interconnected nodes or neurons arranged in layers. These nodes modify their connections according to the computed data. Neural networks are particularly proficient in managing data, which encompasses natural language, audio files, and images.
The necessity of neuro-symbolic artificial intelligence
A substantial challenge is the necessity for neural networks to be more adept at elucidating the relationships between objects. As they rely on readily available data, they are unable to reason. They must acquire common sense.
For example, we have employed neural networks to determine an object’s hue or geometry. Nevertheless, this can be further elaborated upon by utilizing symbolic reasoning to reveal additional intriguing characteristics of the item, including its volume and area.
Symbolic AI systems’ integration of domain knowledge and common-sense reasoning is anticipated to be beneficial.
For instance, a neuro-symbolic system would employ the pattern recognition capabilities of a neural network to identify objects and the logic of symbolic AI to comprehend a shape more effectively during detection.
Neuro-symbolic AI is not exclusively applicable to large-scale models; it can also be effectively implemented with substantially smaller models.
Neuro-symbolic AI has the potential to revolutionize a wide range of applications, from enhancing decision-making processes to deepening our understanding of linguistic nuances. This promising potential opens up new possibilities and sets the stage for a future where AI plays a more significant role in our daily lives, sparking optimism and excitement in the audience.
Neuro-Symbolic AI Integration Methods:
Numerous approaches exist to integrate these two methodologies. Networks are a common approach for processing data and extracting features that are then integrated into a symbolic reasoning system. Another method entails incorporating knowledge into the network’s architecture, which enables it to engage in reasoning during the learning phase.
This is predicated on the contents of Daniel Kahneman’s book, Thinking Fast and Slow.
It is asserted that cognition is divided into two components: System 1, which is reflexive, intuitive, unconscious, and swift.
System 2 is explicit, step-by-step, and ponderous.
System 1 implements pattern recognition.
Deliberative thinking, deduction, and planning are the responsibilities of System 2.
Symbolic reasoning is the most effective method for the second form of cognition, according to this perspective, whereas deep learning is the most effective for the first.
Both are essential for a reliable, robust AI to learn, reason, and interact with humans to accept advice and respond to inquiries. Since the 1990s, numerous researchers have created dual-process models that explicitly reference the two contrasting systems in AI and Cognitive Science.
Beginnings of Neuro-Symbolic Artificial Intelligence
The genesis of Neuro Symbolic AI can be traced back to the intelligence era.
A period of early AI exploration that concentrated on symbolic reasoning was known as the Symbolic Period, which lasted from the 1950s to the 1980s. Systems such as the General Problem Solver and Logic Theorist were created to simulate the problem-solving abilities of humans. To fulfill their obligations, these systems implemented logical reasoning and regulations. She encountered challenges due to the extensive knowledge base required and the inherent variability of the real world.
The 1980s and 2010s: The emergence of neural networks, a resurgence in networks spurred by advancements in computational capabilities and algorithms, redirected AI research toward data-centric approaches. They managed complex tasks and extensive datasets by utilizing techniques such as backpropagation, which enhanced network training.
Nevertheless, neural networks frequently encounter interpretability challenges. Issues arise when obligations necessitate analytical thinking.
From the 2010s to the present, there has been a growing interest in integrating the benefits of neural approaches. Researchers have devised frameworks and models that incorporate networks and reasoning to establish more comprehensible and resilient AI systems. This combination’s objective is to resolve each approach’s deficiencies while leveraging their strengths.
Neuro-Symbolic AI’s primary objectives include:
- Address issues that are even more intricate
- Ultimately, acquire the ability to complete various tasks with significantly less data than a specific task.
- Adopt judgments and behaviors that are both comprehensible and within your capabilities.
- Today’s AI systems require immense data to be trained. AI engineers are required to input thousands of examples into an AI algorithm, whereas a human brain can learn from a few examples.
Neuro-symbolic AI systems can be trained with only 1% of the data required for other methods.
- The development of autonomous systems that can complete tasks without external input is of paramount significance in critical situations such as industrial incidents or natural disasters, and neurosymbolic AI research has the potential to assist in this endeavor.
Neuro-Symbolic AI is a technology that integrates AI’s logic and rule-based systems with networks’ data-driven learning processes.
The primary components of a neuro-symbolic AI system are as follows:
- Artificial neural network
- Symbolic Reasoning Engine
- Integration Layer: This component integrates the symbolic reasoning engine and neural network to establish a hybrid architecture. It facilitates communication between the two elements by mapping the symbolic and neural representations.
- Database of Information
- Generator of Explanation
- User Interface: A component that allows human users to input data and receive output from the neuro-symbolic AI system.
Neuro-Symbolic Artificial Intelligence Applications
Neuro-symbolic AI is implemented in a variety of sectors, such as:
- Neuro-symbolic AI enhances natural language processing (NLP) tasks, including machine translation, information extraction, and question answering, by amalgamating the logical reasoning capabilities of symbolic AI with the comprehension of neural networks.
- Healthcare: Neuro Symbolic AI can offer more precise and interpretable recommendations by incorporating patient data, medical knowledge, and logical reasoning in the context of medical diagnosis and treatment planning.
Neuro-symbolic AI integrates medical expertise with data to facilitate disease diagnosis. It assists in the development of treatment plans by considering the patient’s medical history, current health status, and medical guidelines to provide readily comprehensible recommendations.
Drug Discovery: Integrating data-driven models and reasoning expedites the drug discovery process. Examining chemical structures and biological pathways aids in the identification of drug candidates.
- Robotics: Neuro-symbolic AI is advantageous to autonomous robotics because it utilizes networks to perceive and understand its surroundings and reasoning for decision-making and action planning.
- Finance: Neuro Symbolic AI can enhance industry fraud detection, risk assessment, and investment strategies by integrating rule-based reasoning with data-driven analysis.
Adaptive learning systems in education employ neuro-symbolic AI to personalize students’ learning experiences by analyzing their data and applying principles and knowledge.
Fraud Detection: Financial organizations integrate rule-based analysis with pattern recognition to identify fraudulent activities using Neuro-Symbolic AI. This approach enhances the accuracy and interpretability of fraud detection systems.
Risk Management: Neuro-symbolic AI enables the development of well-informed decisions by integrating market data, historical trends, and regulatory guidelines to facilitate risk assessment.
Cybersecurity requires the integration of AI’s rule-based reasoning and network-driven pattern recognition to comprehend and mitigate intricate cyber threats.
Neuro-symbolic AI, a development in intelligence, represents the potential to develop AI systems that are more resilient, understandable, and efficient. By incorporating the strengths of symbolic reasoning and networks, this hybrid approach demonstrates the potential to address real-world challenges innovatively.
- Industry: Manufacturing.
Predictive Maintenance: Neuro-Symbolic AI optimizes maintenance schedules and minimizes downtime by predicting equipment failures using sensor data analysis and logical principles.
Quality Assurance ensures that manufacturing processes comply with quality standards and detect defects using image recognition and reasoning.
- Retail Industry:
Retail Commerce: Neuro-symbolic AI is implemented by retail organizations to generate customized product recommendations based on consumer preferences and behaviors. Regulations are enforced to guarantee precision and relevance.
Supply Chain Optimization streamlines supply chains by integrating data from various sources and utilizing reasoning to predict demand, optimize logistics, and manage inventory.
- Sector of Education
Customized Learning Systems: Educational platforms employ Neuro-Symbolic AI to improve the learning experience. By analyzing student data and implementing principles, these systems adjust to the learning approaches and requirements of students.
Neuro Symbolic AI’s Intelligent Tutoring Automation enhances the learning experience by allowing tutoring systems to offer students feedback and guidance.
Future Prospects of Neuro-Symbolic AI
The future of neuro-symbolic AI is promising as it expands into various sectors. Some notable trends and potential advancements are as follows:
Improved Transparency:
With AI systems’ increasing complexity, there is a growing need for interpretability and transparency. Neuro Symbolic AI is on the cusp of becoming a leader in developing AI models that offer coherent justifications for their decisions.
Connecting to the Internet of Things:
Smart Gadgets: The Internet of Things (IoT) generates abundant data from interconnected devices. Neuro-symbolic AI can accomplish this. By analyzing this data, you can facilitate the development of autonomous and intelligent devices.
Human Collaboration and Artificial Intelligence:
Neuro-symbolic AI will improve collaboration between humans and machines by offering insights and recommendations based on data and logic, thereby enhancing decision-making.
Developments in Robotics:
Neuro-symbolic AI will improve robotics by incorporating perception and reasoning capabilities, enabling advanced robots to complete intricate tasks. This will be accomplished by employing Independent Systems.
Promoting the Advancement of Ethical Artificial Intelligence:
Bias Mitigation: Neuro-symbolic AI can assist in mitigating biases in AI systems by enforcing regulations and combining data sources to ensure impartial and equitable outcomes.
Neuro Symbolic AI is poised to impact the artificial intelligence landscape as this technology advances significantly.
To sum up, the discipline has experienced numerous exciting developments, including:
- The ethical development of AI.
- The integration with the Internet of Things (IoT).
- The partnership between humans and artificial intelligence.
- Improved comprehension.
These trends are anticipated to affect its trajectory. Neuro-symbolic AI is equipped to address obstacles and create innovative opportunities in a variety of fields by combining the benefits of symbolic reasoning and networks.
Conclusion
We are integrating symbolic reasoning and neural networks in neuro-symbolic AI, which results in the development of adaptable, interpretable, and robust AI systems that significantly advance intelligence.
It is utilized in various sectors, such as finance, healthcare, manufacturing, and education. It enhances capabilities and offers innovative solutions to complex issues.
You can watch my videos related to technology and fintech at my YouTube channel https://www.youtube.com/@
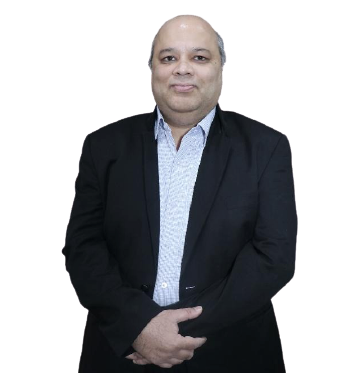